Confidence interval refers to the term that is used in mathematical statistics for the interval estimation of statistical parameters, produced with a small sample size. This interval should cover the value of the unknown parameter with the specified reliability.
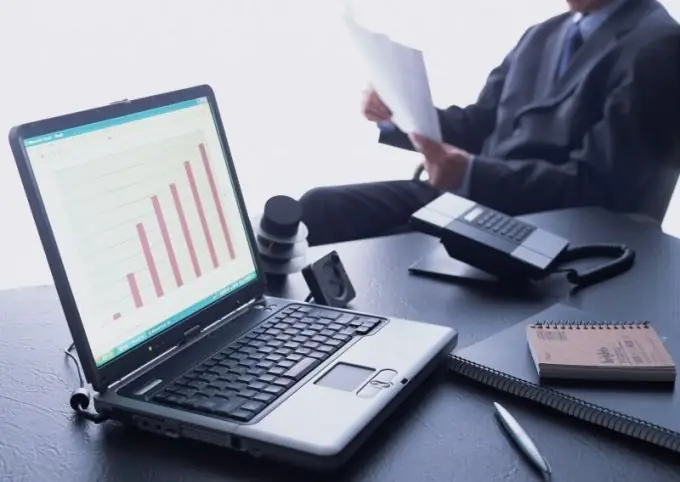
Instructions
Step 1
Note that the interval (l1 or l2), the central area of which will be the estimate l *, and in which the true value of the parameter is enclosed with the alpha probability, will be the confidence interval or the corresponding value of the alpha confidence probability. In this case, l * itself will refer to point estimates. For example, based on the results of any sample values of the random value X {x1, x2, …, xn}, it is necessary to calculate the unknown parameter of the index l, on which the distribution will depend. In this case, obtaining an estimate of a given parameter l * will consist in the fact that for each sample it will be necessary to put a certain value of the parameter in correspondence, that is, to create a function of the observation results of the indicator Q, the value of which will be taken equal to the estimated value of the parameter l * in the form of a formula: l * = Q * (x1, x2,…, xn).
Step 2
Note that any function based on an observation is called statistics. Moreover, if it fully describes the considered parameter (phenomenon), then it is called sufficient statistics. And because the observation results are random, then l * will also be a random variable. The task of calculating statistics should be carried out taking into account the criteria of its quality. Here it is necessary to take into account that the distribution law of the estimate is quite definite if the probability density distribution W (x, l) is known.
Step 3
You can calculate the confidence interval quite simply if you know the distribution law of the estimate. For example, the confidence interval of the estimate in relation to the mathematical expectation (mean value of a random value) mx * = (1 / n) * (x1 + x2 +… + xn). This estimate will be unbiased, that is, the mathematical expectation or average value of the indicator will be equal to the true value of the parameter (M {mx *} = mx).
Step 4
You can establish that the variance of the estimate by the mathematical expectation: bx * ^ 2 = Dx / n. Based on the central limit theorem, we can conclude that the distribution law of this estimate is Gaussian (normal). Therefore, for calculations, you can use the index Ф (z) - the integral of probabilities. In this case, choose the length of the confidence interval 2ld, so you get: alpha = P {mx-ld (using the property of the integral of probabilities by the formula: Ф (-z) = 1- Ф (z)).
Step 5
Plot the confidence interval for the estimate of the expectation: - find the value of the formula (alpha + 1) / 2; - select the value equal to ld / sqrt (Dx / n) from the probability integral table; - take the estimate of the true variance: Dx * = (1 / n) * ((x1 - mx *) ^ 2+ (x2 - mx *) ^ 2 +… + (xn - mx *) ^ 2); - determine ld; - find the confidence interval by the formula: (mx * -ld, mx * + ld).